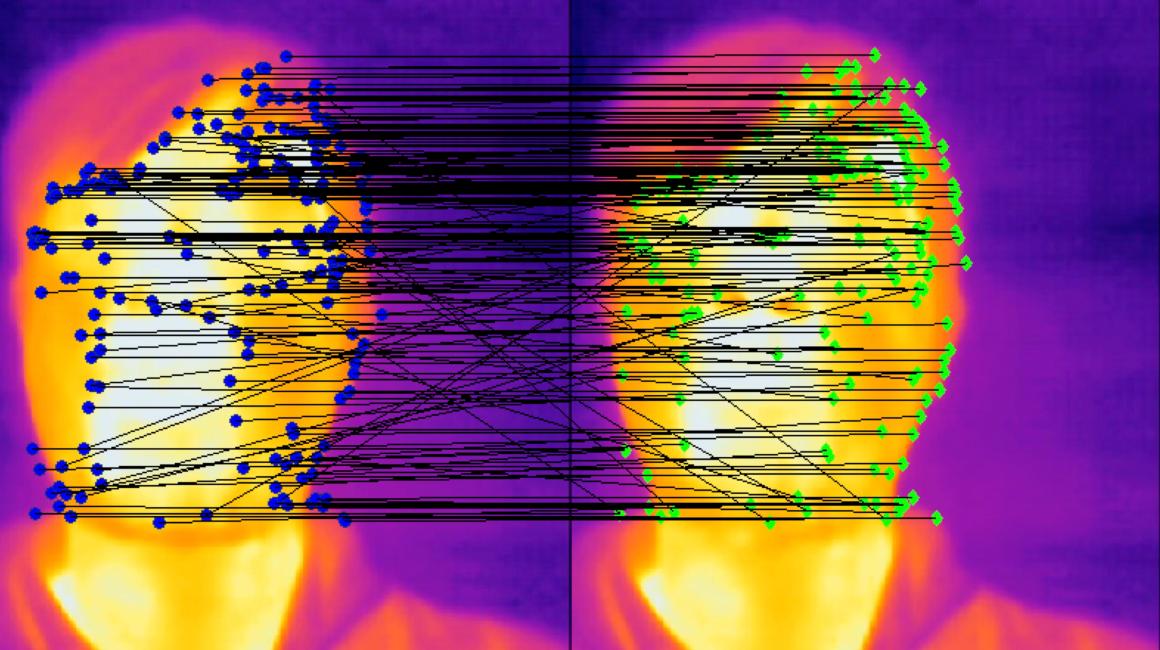
This article was originally published on October 23, 2019.
Chat with UM-Dearborn Assistant Professor of Computer and Information Science Mohamed Abouelenien and you could easily mistake him for a sociologist or psychologist. He counts human behavior — “especially the really interesting stuff” — among his chief research interests. In the past, that’s taken him into fascinating work in lie detection, where he’s built predictive models based on thermal imaging of human faces. And he’s done similar physiological-based research in the field of human alertness, which is hot in the autonomous vehicle world.
One of his latest projects takes aim at a particularly subjective quirk of human physiology: That there seems to be no precise consensus about the temperature at which humans are "thermally comfortable." This becomes especially obvious when we crowd ourselves into shared spaces, like homes, offices or vehicles. As many of us have experienced, the failure of a single temperature setting to keep everyone comfortable is enough to ignite a see-saw battle over the thermostat.
Cars are one place where you've seen more customization, with many models now providing ways for passengers to control their environments semi-independently of the driver. But Abouelenien and his collaborator, UM-Flint Associate Professor of Mechanical Engineering Mihai Burzo, are attempting to take this concept up a few notches. The system they’re working on now would automatically detect each person’s level of “thermal comfort” and then make continuous adjustments to their thermal environment for them.
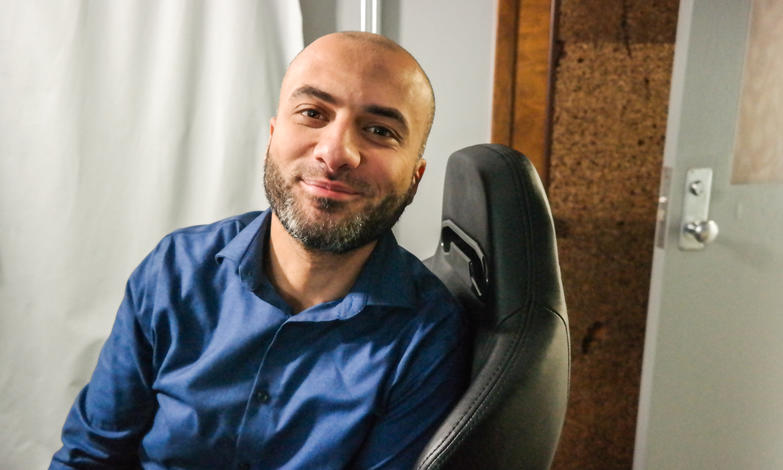
Abouelenien and Burzo started their experiments by collecting some data on thermal discomfort from 50 research subjects. They put them in a closed environment, roughly simulating a vehicle cabin, and then recorded a variety of physiological data under various temperature conditions. A thermal camera, for example, made detailed temperature recordings of their faces, while three other sensors collected information about respiration rate, skin temperature and more than 50 other physiological features. While this was happening, the subjects were also describing their level of thermal comfort under the various conditions. This was important because it would allow Abouelenien and Burzo to correlate those subjective experiences with the sensor information — giving them an idea of what “cold” or “hot” looks like purely from a data perspective.
The team then built a machine learning-based computer model to establish what Abouelenien calls a “decision boundary.” That’s basically a way of defining the Goldilocks zone for a particular person. On one side of this boundary, the model says the physiological sensors indicate a person is likely experiencing cold. On the other, their model indicates a person is likely getting too hot.
They then put their model to the test — running a second trial which, again, exposed research subjects to various conditions and then asked them about their level of thermal comfort. Like before, their systems also tracked physiological data of their experiences.
This time, however, their model was also using the data to make predictions about what the subjects’ responses would be. The results were pretty shocking. In some cases, particularly with cold, their model was making a correct prediction about subjects’ reported experience more than 90 percent of the time. In other words, it knew how they were feeling.
Abouelenien says the obvious debut application for a technology like this would be in a vehicle cabin, where their algorithms could guide an HVAC system that adjusted itself automatically. The advantage of a vehicle setting is that the driver or passenger maintains a relatively consistent position, giving the sensors a stationary target. Plus, there’s a clear benefit beyond mere comfort: Their algorithmically guided thermostat is more efficient than driver controlled ones, and the energy savings could ultimately help improve electric vehicle range.
As for whether you could deploy this technology in a home or office, Abouelenien says we’re not quite there yet. He says some of the main challenges are that people move around a lot in those settings, and the system would therefore require a bigger network of sensors. But he says it’s also not hard to see how it could happen. The price for thermal imaging cameras — the device they’ve found to be the best overall predictor of thermal comfort — has come down a lot. And his next project is looking at ways to collect physiological data using non-contact sensors, which, as the name suggests, don’t have to be physically connected to a person.
Given all that, declaring a cease fire in the battle over the thermostat might just be a matter of time.