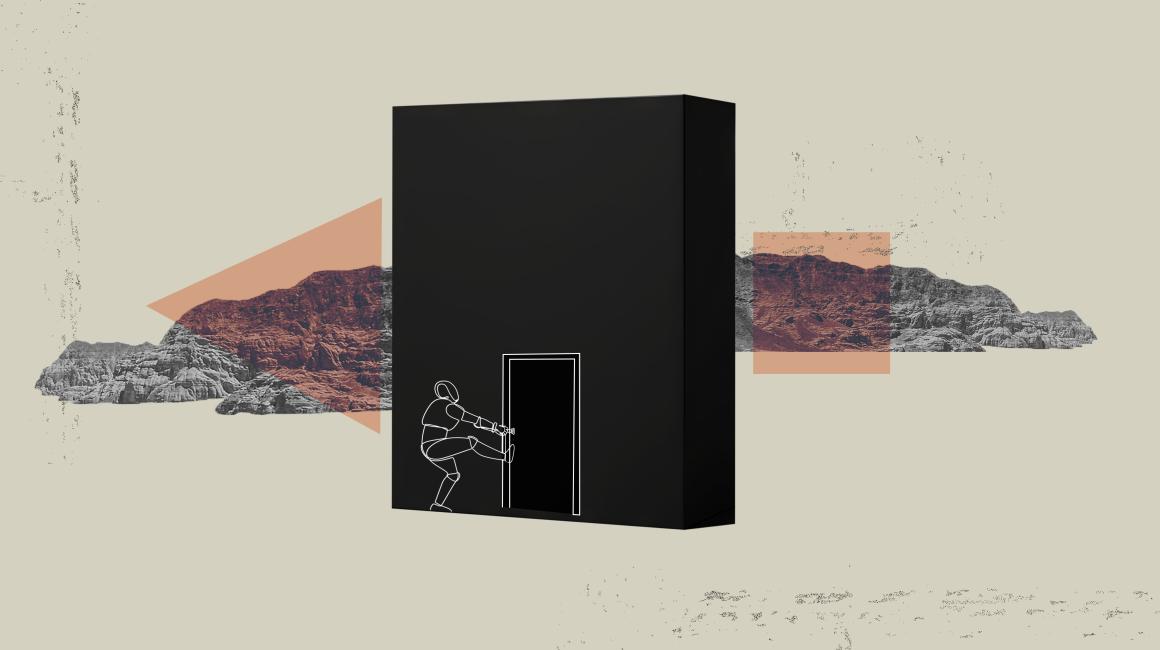
Learning by example is one of the most powerful and mysterious forces driving intelligence, whether you’re talking about humans or machines. Think, for instance, of how children first learn to recognize letters of the alphabet or different animals. You simply have to show them enough examples of the letter B or a cat and before long, they can identify any instance of that letter or animal. The basic theory is that the brain is a trend-finding machine. When it’s exposed to examples, it can identify qualities essential to cat-ness or B-ness, and these ultimately coalesce into decision protocols that give us the ability to categorize new experiences automatically and unconsciously. Doing this is easy. Explaining how we do this is essentially impossible. “It’s one of those weird things that you know, but you don’t know how you know it or where you learned it,” says Associate Professor of Electrical and Computer Engineering Samir Rawashdeh, who specializes in artificial intelligence. “It’s not that you forgot. It’s that you’ve lost track of which inputs taught you what and all you’re left with is the judgments.”
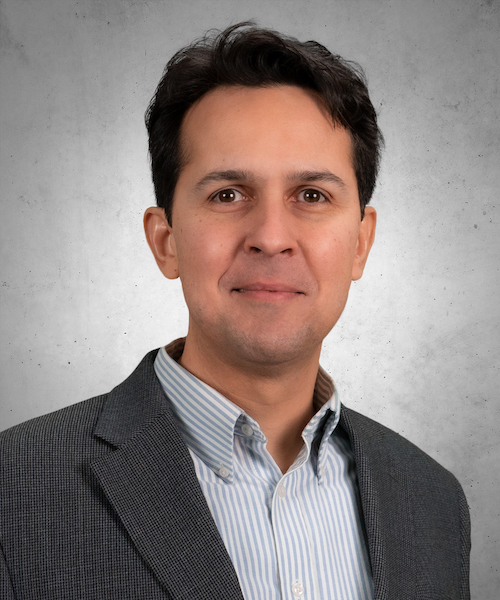
Rawashdeh says deep learning, one of the most ubiquitous modern forms of artificial intelligence, works much the same way, in no small part because it was inspired by this theory of human intelligence. In fact, deep learning algorithms are trained much the same way we teach children. You feed the system correct examples of something you want it to be able to recognize, and before long, its own trend-finding inclinations will have worked out a “neural network” for categorizing things it’s never experienced before. Pop in the keyword “cat” — or even the name of one of your favorite cats — into the search bar of your photo app and you’ll see how good deep learning systems are. But Rawashdeh says that, just like our human intelligence, we have no idea of how a deep learning system comes to its conclusions. It “lost track” of the inputs that informed its decision making a long time ago. Or, more accurately, it was never keeping track.
This inability for us to see how deep learning systems make their decisions is known as the “black box problem,” and it’s a big deal for a couple of different reasons. First, this quality makes it difficult to fix deep learning systems when they produce unwanted outcomes. If, for example, an autonomous vehicle strikes a pedestrian when we’d expect it to hit the brakes, the black box nature of the system means we can’t trace the system’s thought process and see why it made this decision. If this type of accident happened, and it turned out that the perception system missed the pedestrian, Rawashdeh says we’d assume it was because the system encountered something novel in the situation. We’d then try to diagnose what that could have been and expose the system to more of those situations so it would learn to perform better next time. “But the challenge is, can you get training data that covers everything?” Rawashdeh says. “What about when it’s sunny and a bit foggy, or they’ve just salted the roads and the asphalt now appears whiter than it usually does? There are an infinite number of permutations so you never know if the system is robust enough to handle every situation.”
Rawashdeh says this problem of robustness makes it difficult for us to trust deep learning systems when it comes to safety. But he notes the black box problem also has an ethical dimension. Deep learning systems are now regularly used to make judgements about humans in contexts ranging from medical treatments, to who should get approved for a loan, to which applicants should get a job interview. In each of these areas, it’s been demonstrated that AI systems can reflect unwanted biases from our human world. (If you want to know how AI systems can become racially biased, check out our previous story on that topic.) Needless to say, a deep learning system that can deny you a loan or screen you out of the first round of job interviews but can’t explain why, is one most people would have a hard time judging as “fair.”
So what can we do about this black box problem? Rawashdeh says there are essentially two different approaches. One is to pump the brakes on the use of deep learning in high-stakes applications. For example, the European Union is now creating a regulatory framework, which sorts potential applications into risk categories. This could prohibit the use of deep learning systems in areas where the potential for harm is high, like finance and criminal justice, while allowing their use in lower-stakes applications like chatbots, spam filters, search and video games. The second approach is to find a way to peer into the box. Rawashdeh says so-called “explainable AI” is still very much an emerging field, but computer scientists have some interesting ideas about how to make deep learning more transparent, and thus fixable and accountable. “There are different models for how to do this, but we essentially need a way to figure out which inputs are causing what,” he says. “It may involve classical data science methods that look for correlations. Or it may involve bigger neural nets, or neural nets with side tasks, so we can create data visualizations that would give you some insight into where the decision came from. Either way, it’s more work, and it’s very much an unsolved problem right now.”
At the end of the day, the question of what role AI should play in our lives may not be fundamentally different from the conversations we have anytime a potentially transformative technology emerges. Typically, that conversation involves a calculation of risks and benefits, and Rawashdeh thinks it’s still early enough for us to have thoughtful conversations about how and how quickly we want deep learning to shape our world. “Without question, there is a huge potential for AI, but it gets scary when you get into areas like autonomy or health care or national defense. You realize we have to get this right. For example, whenever I have a moment when I’m disconnected from the internet for a few days, I'm reminded just how different that reality is than the modern reality that’s shaped by social media or all the things we immerse ourselves in online. When the internet came into being, we just let it into our world, and in hindsight, we can see that came with certain risks. If we could turn back the clock 30 years, knowing what we know now, would we just let the internet loose on people? I think it’s a similar decision that we face now with AI.”
###
Story by Lou Blouin