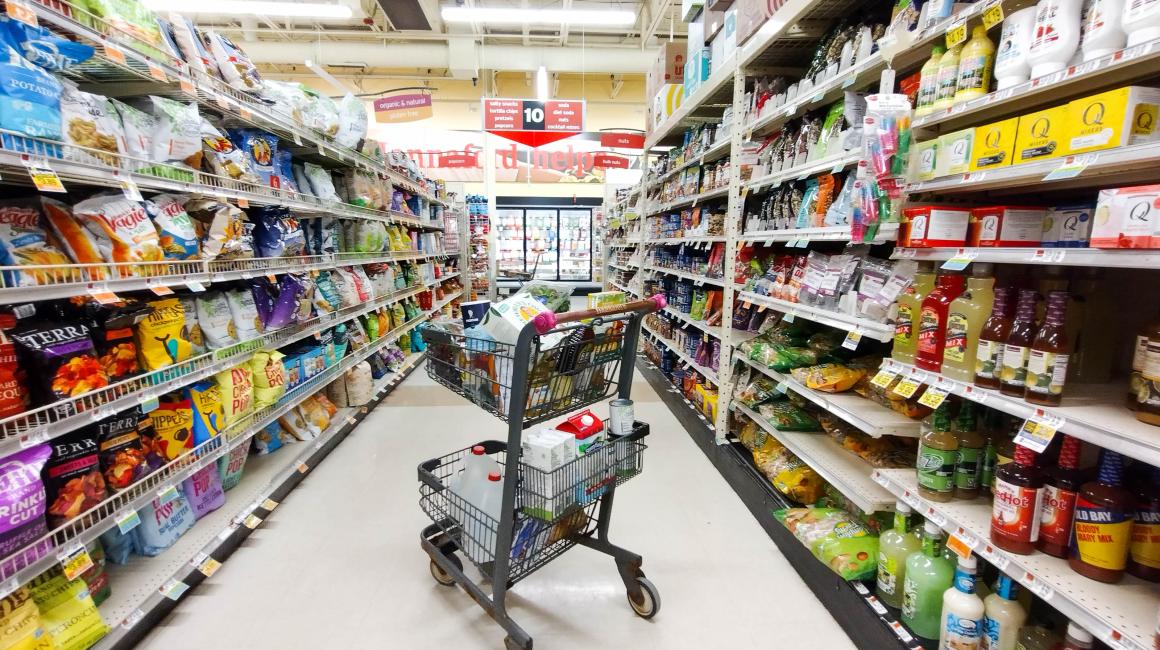
How does the rice you buy at the grocery store stay shelf stable basically indefinitely? Or why can you expect your favorite crackers will always have the same color, taste and crispness — despite being made of always slightly varying batches of flour, cheese and oil? We owe a lot of the longevity and predictably of the food supply to canning, drying and dozens of other technologies and processes that are collectively known as “food processing.” Yet surprisingly, this consistency is earned through a fair amount of inefficient trial and error on the farm or factory floor, according to UM-Dearborn Associate Professor of Industrial and Manufacturing Systems Engineering Cheol Lee. In a potato chip factory, for example, Lee says an inspector might notice the chips coming off the line today are a little too brown, so they try cooking the next batch for 10 seconds less. Still too brown. So they try 20 seconds less. Just right! But then, tomorrow’s batch, which is made using potatoes from a different farm, isn’t brown enough. So they adjust the cooking time again. “Obviously, this is not an optimal way to do it. You waste a lot of energy and time and reduce yields,” Lee says.
Finding a more efficient way to make these process adjustments is the subject of new research led by Lee in collaboration with Professor of Mechanical Engineering Oleg Zikanov and partners at Michigan State University. Their project, funded by a $1.2 million grant from the U.S. Department of Agriculture — UM-Dearborn’s first from the agency — focuses on optimizing food drying processes, which are some of the most energy intensive in the industry. Lee and Zikanov’s hypothesis is that many of the tweaks that humans make now through educated guesswork could be better executed by a computer model — specifically, a high-fidelity physics-based model. The basic idea is that the dynamics inside the drying equipment, like the way air moves or heat is transferred, are subject to the basic principles of physics. And if you could build a mathematical model that described all of those dynamics, you could actually predict the output of the drying process — e.g. color, crispiness, bacteria levels — from some easy-to-measure inputs, like the moisture content of the pre-processed food, drying temperature, fan speed, speed of the conveyor, etc. Thus, with a good model, you could eliminate the need for human trial-and-error adjustments — and the wasted food, energy and carbon emissions that come with that.
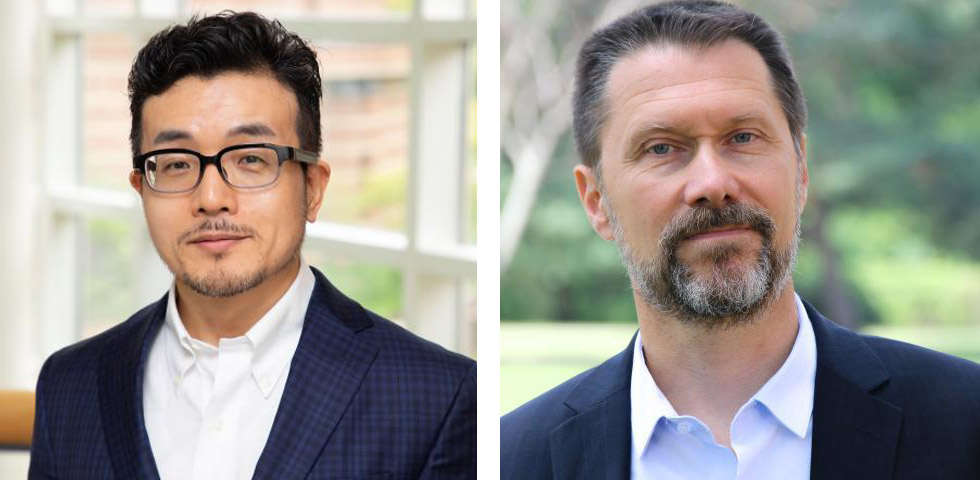
Modeling highly complex phenomena like this is possible, but this approach typically has a major drawback: For the models to be very accurate without having to resort to trial and error themselves, they have to account for so many variables and relationships between variables that they become very large, and thus very computationally intensive. “It can take days, and sometimes weeks, to simulate one possible scenario, which is not good when you’re talking about processing conditions changing possibly every couple of hours,” Lee explains. “So you need a way to simulate the process a lot faster.” Their solution is an approach called reduced order modeling, which can drastically shrink the size of the model — thus making it speedier — while sacrificing very little accuracy, a bit like the way image compression creates photos that look nearly identical to the originals, but at a much smaller file size. “The basic philosophy is that you have an output that is very high dimensional and contains a lot of information,” Lee explains. “But most of the solutions lie in a very small subset of this very large space. We call that subspace. And the challenge is, how do we find this subspace where the solution can exist?”
Lee and Zikanov have special techniques for finding this subspace, and they’ve actually used this approach successfully on two other recent projects. One modeled how the coronavirus could spread throughout an indoor environment. The other, a collaboration with General Motors, created a reduced order model for cooling lithium ion batteries in electric vehicles. Despite the drastically reduced size and increased speed of the models, Lee says they still had more than 99 percent of the accuracy of the original model — meaning a modest computer could execute the smaller version in seconds or less, compared to the days or weeks needed for the computationally heavy models. Another advantage of this approach: It’s light on hardware. In a food processing environment, Lee says it would integrate easily into factory equipment with a few controllers and draw on information that existing sensors are already collecting.
Throughout the course of the three-year project, Lee and Zikanov will also field test their model and control algorithm on actual drying equipment located at Michigan State University. “If everything goes well, we’ll end up with a model that can, in real time, make predictions about what is going to happen, then take in data from a few sensors and optimize the process on the go. That’s the bright future for this technology,” Zikanov says. Plus, because drying is a common process in many industries, from pulp production to pharmaceuticals, Lee says the technology could have a wide range of applications.
One other cool part of their project: In an effort to get young people interested in STEM subjects, Lee and Zikanov will periodically host high school students from University Prep Academy High School in Detroit, who will also get to tour the drying facilities at Michigan State. Lee and Zikanov’s research team will also include several UM-Dearborn pre-engineering students. These are students who plan on entering engineering programs but are still working on meeting their introductory math and science requirements. Lee and Zikanov are hoping the hands-on learning from this project will help get students over the hump and into their chosen programs in the College of Engineering and Computer Science.
###
Lee and Zikanov’s project is funded through a U.S. Department of Agriculture and National Science Foundation interagency program. Story by Lou Blouin