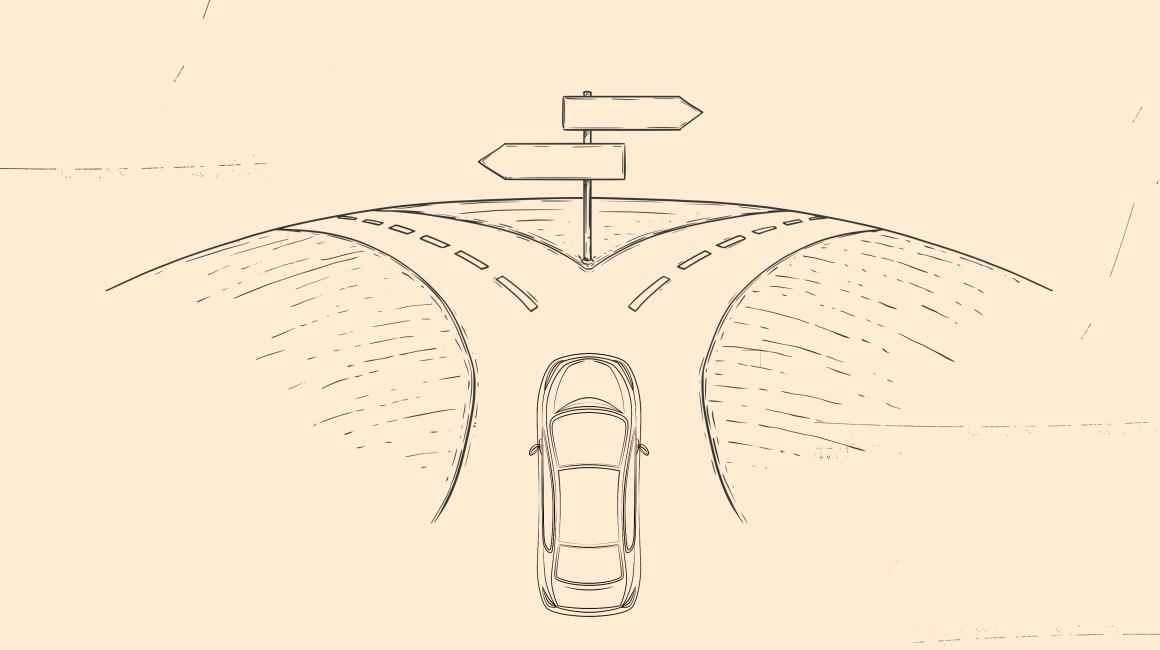
Not every conversation with engineering faculty starts with a presentation slide featuring 17th-century thinker René Descartes and his signature view of dualism, a philosophy that placed the mind distinct from, and in a hierarchical relationship to, the body. Assistant Professor of Electrical and Computer Engineering Jaerock Kwon, however, is not your typical engineering professor. After starting his career as a software engineer, he completed his doctoral work in computational neuroscience, a field where researchers use mathematical models and computer simulations to understand the development of our cognitive processes. His latest work — “embodied cognitive approaches to autonomous vehicles” — is a great example of his penchant for the unconventional, and the project is as weird and fascinating as it sounds. In a nutshell, Kwon thinks it’s possible that some of the challenges we’ve run into trying to create fully autonomous vehicles stem from the fact that we’ve been thinking about AVs too dualistically. His hypothesis: To make big breakthroughs in autonomy, maybe we shouldn’t be thinking about driverless vehicles just as big computers that control a physical car, but as embodied cognitive beings that have intuitive understanding of how to control their physical presence in the world. Sort of like us.
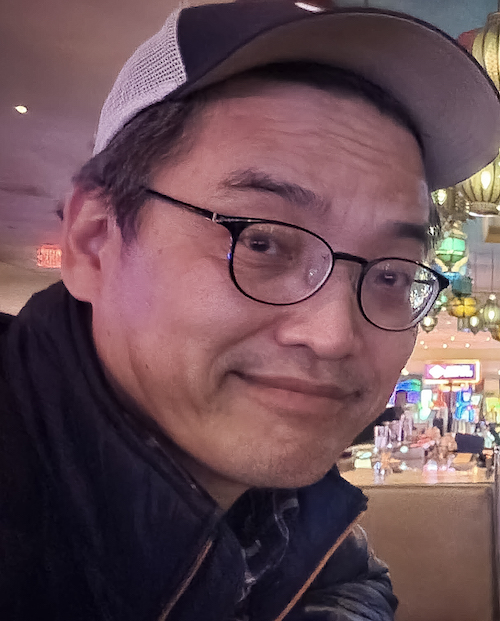
To understand what exactly Kwon means by this, it helps to understand the paradigm that dominates autonomous vehicle research today. Current experimental AVs are outfitted with lots of sensors that allow them to experience their environment, and powerful computing units then interpret that sensor data and relay decisions about how to act to the mechanical parts of the car. A core part of the vehicle’s intelligence comes from machine learning processes that apply labels to relevant parts of its sensed environment. For example, if the computer interprets an image provided by the vehicle’s optical cameras as containing a “traffic signal with a red light,” it will then send a signal to apply a proper amount of pressure to the brakes in order to come to a stop. It sounds simple enough. But an action like that might involve dozens and dozens of coordinated computational processes that take into account dozens more variables, like distance to the traffic light, the distance to a car already stopped at the light, the speed and weight of the vehicle, its center of gravity, the amount of friction between the tires and the road, and so on. Now imagine programming a car not just for stopping at a red light but for all the situations it might encounter — including unpredictable so-called “edge cases,” like interactions with pedestrians or aggressive drivers. It's easy to see that this inherent complexity is a big reason why experts think we could still be decades away from driverless cars hitting the market.
For Kwon, something about this approach always seemed a little strange. Essentially, we’re trying to recreate a set of human behaviors — namely, good driving — but the process we’ve devised doesn’t really resemble at all how we drive. “Think about when you first learned how to drive,” he says. “You weren’t making a bunch of mathematical calculations. All you did was start doing things, and then you adjusted your behavior based on what happened so you could make the car do what you wanted it to do.” Put another way, we were learning by “motor babbling.” We were simply trying out different actions and then gradually refining those actions based on what we perceived. We learned how to have the right touch with the accelerator by first hitting the gas too hard and peeling out. We learned to lane keep by experiencing what it feels like to turn the wheel too hard and drift toward the shoulder or centerline.
This behavior, Kwon argues, can’t be described by a dualistic model in which the brain is simply processing a bunch of sensory information, measuring it, categorizing it, “solving a bunch of differential equations,” and then sending action directives to the body. Rather, it’s an embodied cognitive process in which our sensory perception and our motor coordination have become more directly and intuitively connected through a series of shared experiences. If we accept this, it's a natural next question to consider whether you could get a car to drive by simply allowing it to learn, much like we do. Within certain parameters, the answer actually is yes. Though Kwon’s work may sound far out, artificial intelligence researchers have been down this path more than once. In the late 1980s, robotics researchers at Carnegie Mellon University adapted a U.S. Army ambulance for autonomous driving that was powered by a brand of artificial intelligence called behavior cloning. Basically, the vehicle observed how humans drove, and after thousands of hours, it was able to recognize the relevant features of the environment that caused humans to react the way they did. It even worked well enough to make supervised interstate trips between Pittsburgh and Erie, Pa. Fast forward to 2016 and NVIDIA, the artificial intelligence and graphics processing unit company, used a similar method to create a deep learning vehicle that at first drove like an intoxicated driver. But after just 3,000 miles, it could lane keep quite well, even when lane markers weren’t present.