
The workforce that powers American farms is facing stress from many directions. The average age of the country’s farmers has climbed steadily since the 1970s and now stands at 57-and-a-half years old — less than a decade shy of the Social Security retirement age. Young people, staring down the prospect of low wages, high stress and problems accessing land, aren’t rushing in to replace older farmers. For the past several years, farms have also had a hard time finding enough workers, as fewer people come to the U.S. for seasonal agricultural employment and veteran farmworkers age out of the profession. Meanwhile, a huge majority of Americans continue to show little interest in doing agricultural labor, which is hard on your body, doesn’t pay particularly well and can expose you to harmful pesticides.
Potential solutions to the labor shortage come in a variety of forms, from immigration reform to grants that help a younger, more diverse group of Americans buy increasingly expensive farmland. Assistant Professor of Electrical and Computer Engineering Jaerock Kwon thinks robots could also help ease farms’ labor challenges — particularly common sense robots that amplify the impact of human farmworkers by helping with straightforward tasks. Viewed in terms of modern agricultural history, it’s really not such a radical idea. Over the past hundred years, the number of people working on American farms has declined drastically, while yields have increased and the amount of farmland in production has decreased. The reason? We shifted work to machines like tractors, combines and food processing technology, though human labor is still needed for many tasks on both big and small farms. In particular, people will likely still be needed for the foreseeable future to harvest crops requiring gentle handling. But Kwon thinks less complicated tasks, like toting bushels of apples through an orchard or applying pesticides or fertilizers, are well within a robot’s capabilities.
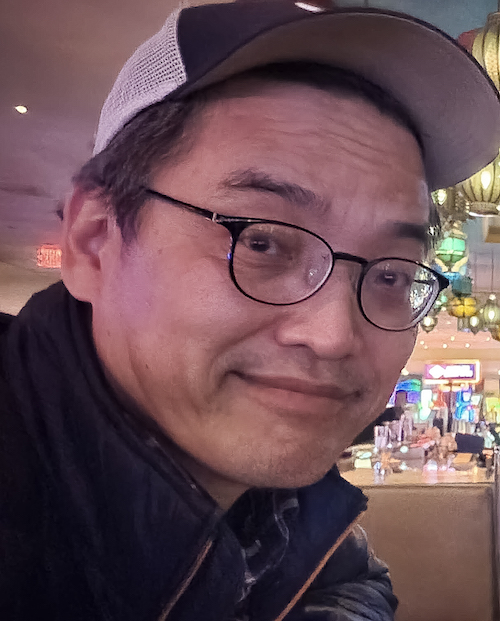
Kwon thinks some of the most practical robots for the farm, at least right now, are likely autonomous vehicles. But designing AVs for farmwork presents a much different challenge than those designed for the road or an indoor environment like a warehouse. For example, Kwon says that AVs typically keep track of where they are in space with the help of motion sensors, which measure the distance the vehicle has traveled. But this system’s programming typically assumes a flat surface. On uneven surfaces, like a farm field, the distance that an AV’s wheels travel along the ground will be greater than the as-the-crow-flies distance between two horizontal points, owing to bumps and dips in the terrain. One wheel might even travel a different distance than the one next to it. And Kwon says these little deviations could cause a traditional AV’s motion sensors to guide the vehicle off course.
Similarly, a traditional AV’s vision system might leave it totally lost on the farm. Many AVs have to map their environment first, which helps the vehicle identify important landmarks and keep track of where it is. “But if you look at, say, an orchard environment, with rows and rows of evenly spaced trees, there are no distinguishing characteristics. It all looks the same,” Kwon explains. “The robot would look around and have no idea where it was.” Kwon says the variable lighting of outdoor conditions could also confuse AVs’ optical cameras and image recognition systems, which often rely on properties like color to identify objects. A shiny red object may always look the same in a windowless warehouse. However, in the orchard, the object might appear red under cloudy skies at noon, but white if it’s reflecting bright sunlight. At sunset, it might look sort of rust-colored.
Kwon thinks fixing these individual problems with ever more complex programming — how we’ve traditionally fine-tuned AVs — isn’t the best option. Instead, he thinks farm AVs will work much better if we take a fundamentally different approach. Specifically, he thinks farm vehicles could be a great application of a concept he’s been working on called embodied cognitive driving. Typical AVs have sensors to experience their environment, and then, using a bunch of very complicated math, their powerful computing units interpret that sensor data and relay decisions about how to act to the mechanical parts of the vehicle. An embodied cognitive AV is conceptually and computationally much simpler. Using machine learning, it learns to navigate an environment simply by observing how a human driver does it first, deriving its own rules for, say, accurate steering on uneven ground. It doesn’t even have an image recognition system to do things like recognize what an apple tree is, nor programming that tells it how to not run into one. Kwon’s vehicle wouldn’t even know what an apple tree is, and it doesn’t need to. It simply learns to avoid such objects because that’s what it’s always seen its human mentor do.
This may sound like magic, but there are actually examples of machine learning-based vehicles like this learning to be pretty good drivers dating back to the 1980s. And Kwon thinks this approach, which has fallen out of favor among engineers creating driverless cars, could actually work much better for farm AVs. Traditional AVs, with their powerful interpretive capacities, would certainly outperform Kwon’s farm AV on city streets. In that environment, the traditional AV’s programming would allow it to recognize people, stop signs and lane markers — useful abilities for safe navigation on the road. Trained in the orchard, Kwon’s vehicles likely wouldn’t know how to react to such objects. But in the orchard, the traditional AV would be the one getting confused, because the environment is simply too irregular for its brand of intelligence to work very well. Meanwhile, Kwon’s vehicle would navigate the orchard rows, as humans do, with unconscious, thoughtless ease, because its motor skills have specifically evolved to move in the bumpy, irregular terrain. “They don’t have to know what they’re doing,” Kwon says. “They just need to do their jobs well.”
Kwon also notes that farmwork is full of straightforward tasks that this computationally lighter style of AV could master pretty quickly. To return to the orchard example, as a person picks apples, they collect them into bags worn on their bodies, and then they dump those bags into crates. But what if a human worker had a small, lightweight AV helper, which simply trailed them with a container for collecting the apples? That would save work for the human laborer — and relieve the burden of carrying heavy bags around, a break the country's aging farmers and farmworkers could no doubt use. In fact, Kwon says one of the first tasks they’ll be testing with their new prototype vehicle is how well it can follow a human over uneven ground. “I don’t have an orchard, though,” Kwon says, smiling. “So don't be surprised if you see one of my graduate students walking around campus with a robot following them this fall.”
###
Want to learn more about Kwon’s research on embodied cognitive AVs? Check out our story, “Have we been thinking about autonomous vehicles all wrong?” Story by Lou Blouin